About
I am a postdoctoral research scholar in the Decision, Risk, and Operations Division at Columbia Business School, working with Hongseok Namkoong and Assaf Zeevi. I earned my PhD in Management Science and Engineering from Stanford University, where I had the privilege of being advised by Peter Glynn and Jose Blanchet. I completed my bachelor's degree in Mathematics at the University of Science and Technology of China (USTC).
Research
I am interested in leveraging scalable tools to analyze complex systems including stochastic models in operations research and stochastic algorithms in machine learning. To facilitate this analysis in great generality, I develop theories and algorithms in the realm of general state-space Markov chains, as summarized in my thesis Markov Chain Convergence Analysis: From Pen and Paper to Deep Learning.
Deep Learning for Computing Convergence Rates of Markov Chains
with Jose Blanchet and Peter Glynn, NeurIPS (spotlight), 2024
Computable Bounds on Convergence of Markov Chains in Wasserstein Distance
with Jose Blanchet and Peter Glynn, Annals of Applied Probability, accepted, 2025
- Applied Probability Society Best Student Paper Prize, 2023
- Applied Probability Society Conference Best Poster Award, 2023
Rubik's Cube Scrambling Requires at Least 26 Random Moves
On a New Characterization of Harris Recurrence for Markov Chains and Processes
with Peter Glynn, Mathematics, 2023
Strong Limit Interchange Property of a Sequence of Markov Processes
with Jose Blanchet and Peter Glynn, preprint
Estimating the Convergence Rate to Equilibrium of a Markov Chain via Simulation
with Jose Blanchet and Peter Glynn, preprint
Bias of Markov Chain Sample Quantile
with Peter Glynn, preprint
Uniform Edgeworth Expansion for Markov Chains
with Peter Glynn, preprint
I am currently continuing my work on Markov chains while also exploring a new direction in multi-armed bandits.
What does Thompson Sampling Optimize?
with Hongseok Namkoong and Assaf Zeevi, ongoing work
We rediscover Thompson Sampling as an online optimization algorithm that minimizes immediate squared regret adaptively regularized by an uncertainty measure.
Non-compact Deep Contractive Drift Calculator
with Jose Blanchet and Peter Glynn, ongoing work
We automate the convergence analysis of Markov chains on non-compact state spaces by reducing the task to function approximation (via neural networks) on compact sets.
Teaching
Centennial Teaching Assistant for the following MS&E courses at Stanford
Contact
qu dot yanlin at columbia dot edu
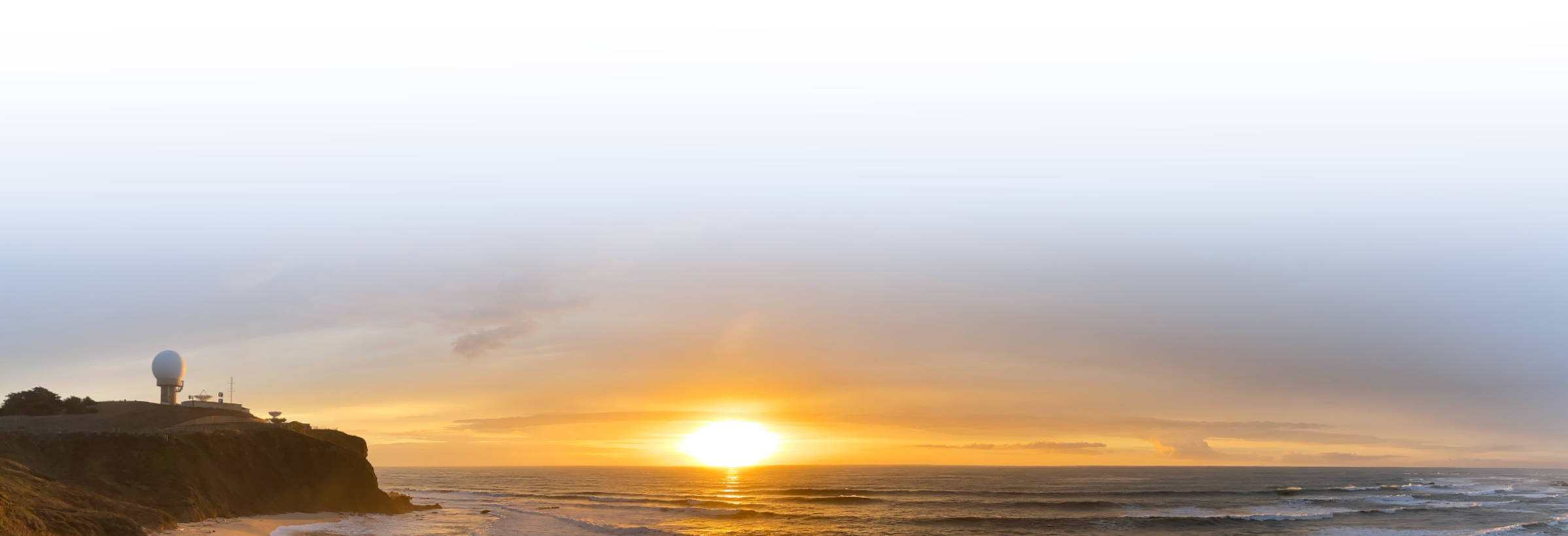