This event has been supported financially by donations from Cardinal Operations, MOSEK, the Stanford Department of Management Science and Engineering (MS&E), the Stanford MS&E Operations Research Group, Yichuan Ding, Jiawei Zhang, and Zeyu Zheng. In addition, the organizers wish to thank our MS&E staff, Jackie Nguyen and Roz Morf, for their tireless efforts in supporting the organization and running of this activity, and to Tim Keely for his IT assistance. Additionally, the following PhD students have played a central role in helping support this activity: Sirui Lin, Hao Liu, Yanlin Qu, Haoran Xu, Wenhao Yang, Tony Ye, and Jiacheng Zou.
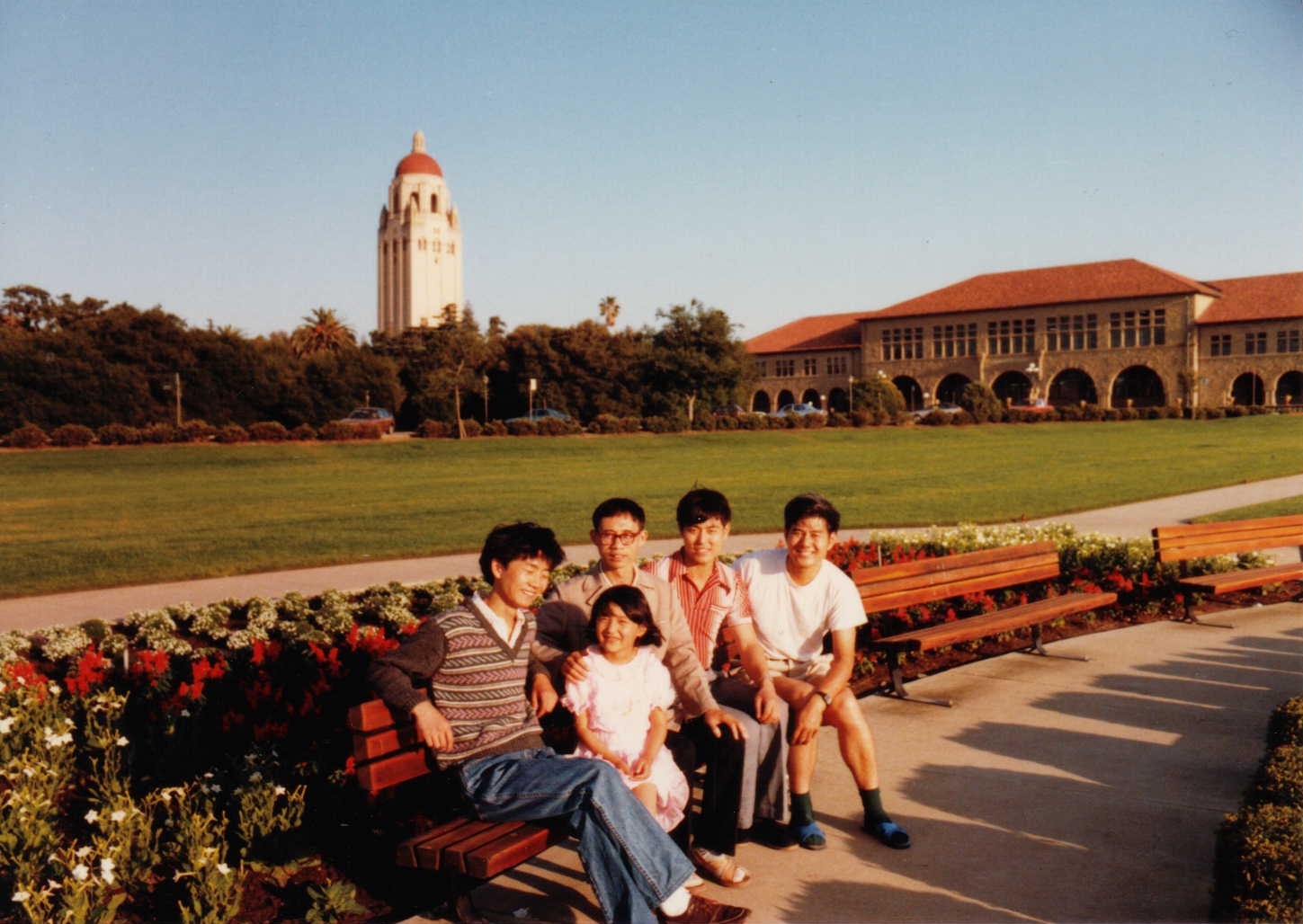
Yinyu Ye
Retirement Celebration
July 28-29, 2024, Stanford
About The Event
We honor and celebrate the remarkable career of Professor Yinyu Ye. As a distinguished scholar in the field of optimization, Professor Ye has made significant contributions that have advanced both the theory and the practice of optimization. This two-day event will feature a series of talks from Professor Ye's students and collaborators.
Where
Hewlett Teaching Center, Room 201, Stanford University
When
July 28-29, 2024 (Sunday-Monday)
Event Schedule
Lunch Tresidder Union
Opening Remarks
Session 1 (Chair: Yichuan Daniel Ding)
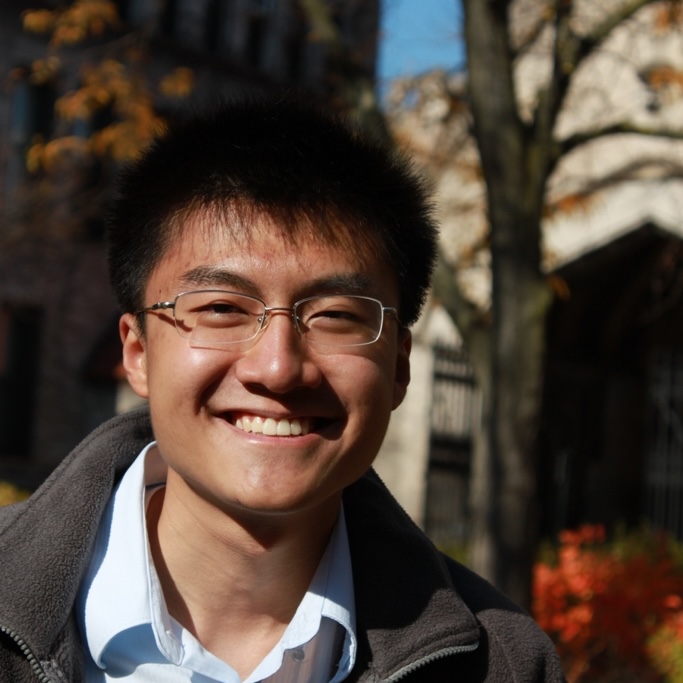
Zizhuo Wang CUHK, Shenzhen
Learning and Working with Prof. Yinyu Ye
 
In this talk, I would share some of my experiences and stories learning and working with Prof. Yinyu Ye, who have greatly guided my professional career.
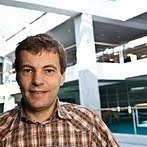
Erling Andersen MOSEK
Basis Identification in Linear Optimization Revisited
 
Basis identification is the problem of computing an optimal basic (vertex) solution to an linear optimization problem given any primal-dual optimal (non vertex)solution. This is an important problem in practice since interior-point methods do not produce an optimal basic solution in general and therefore a basis identification procedure may be required.In this presentation we will review the joint work by Yinyu Ye and the speaker on basis identification. Finally, subsequent work on practical issues related to implementing a basis identification procedure is discussed.
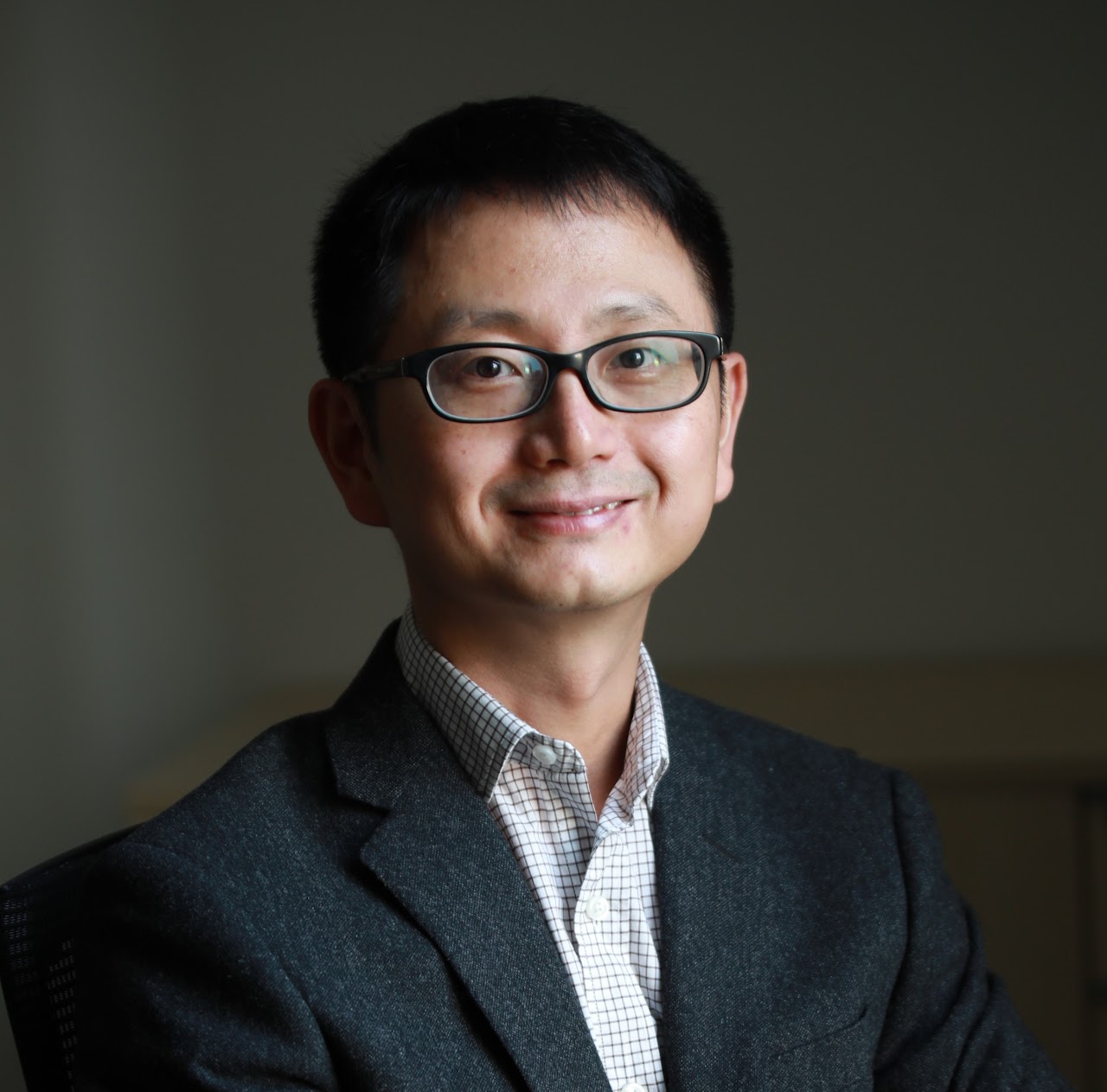
Jiawei Zhang New York University
A Primal-Dual Algorithm for Nonstationary Online Stochastic Optimization
 
We consider a general online stochastic optimization problem with multiple budget constraints over a horizon of finite time periods. In each time period, a reward function and multiple cost functions are revealed, where cost function corresponds to the consumption of one budget constraint, and the decision maker needs to specify an action to collect the reward and consume the budgets. The objective of the decision maker is to maximize the cumulative reward subject to the budget constraints. This formulation captures a wide range of applications including online order fulfillment and network revenue management, among others. The reward function and the cost functions of each time period are drawn from a distribution that could be arbitrarily non-stationary. We develop an informative gradient descent algorithm, which integrates the non-stationary distributional information into an online gradient descent procedure. This is a joint work with Jiashuo Jiang (HKUST) and Xiaocheng Li (ICL).
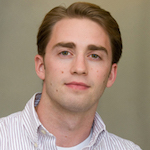
John Carlsson University of Southern California
15 Years of Ham Sandwiches in Logistics
 
The ham sandwich theorem is a famous result of algebraic topology and computational geometry that states that, given \(n\) measures in \(\mathbb{R}^n\), there exist a single \((n-1)\)-dimensional hyperplane that simultaneously divides all of them in half. By combining this result with results from the continuous approximation paradigm in logistics, it is possible to construct provably optimal partitions of a service region for the purpose of assigning service districts to vehicles. This talk presents various discoveries in this area from the last 15 years.
Coffee Break
Session 2 (Chair: Aaron Sidford)
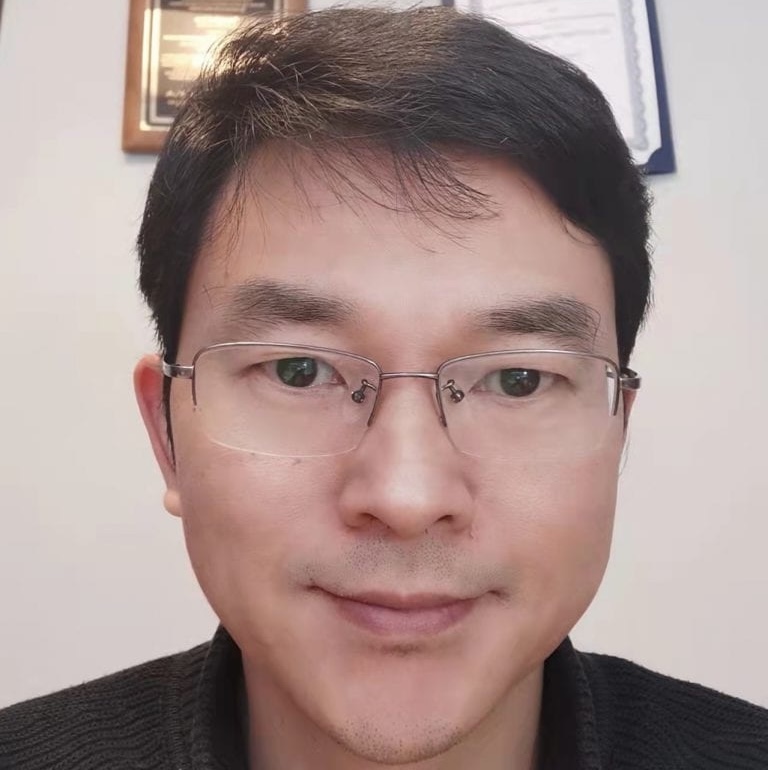
Guanghui Lan Georgia Institute of Technology
Policy Mirror Descent for Reinforcement Learning
 
Markov decision processes and reinforcement learning (RL) have been studied from various perspectives, including linear programming and dynamic optimization. In this talk, we will discuss some recent advances that bridge RL with stochastic nonlinear programming. Specifically, we will introduce a new and general class of policy mirror descent (PMD) methods, demonstrating that they achieve linear convergence in deterministic cases and optimal sampling complexity in stochastic cases for discounted Markov decision processes. We will also explore extensions of these algorithms under different settings.
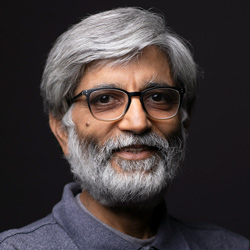
Sanjay Mehrotra Northwestern University
On Approximation of Robust Max-Cut and Related Problems using Randomized Rounding Algorithms
 
The randomized rounding algorithm for the Max-Cut problem achieves a 0.878 approximation bound in expectation while solving a semidefinite program. The 0.878 approximation bound remains the best-known approximation bound for this APX-hard problem. Their approach was subsequently applied to other related problems such as Max-DiCut, Max-SAT, and Max-2SAT, etc. We show that the randomized rounding algorithm can also achieve a 0.878 approximation bound for the robust and distributionally robust counterparts of the max-cut problem. Approximation bounds are maintained for the robust and distributionally robust counterparts of other problems are also maintained.
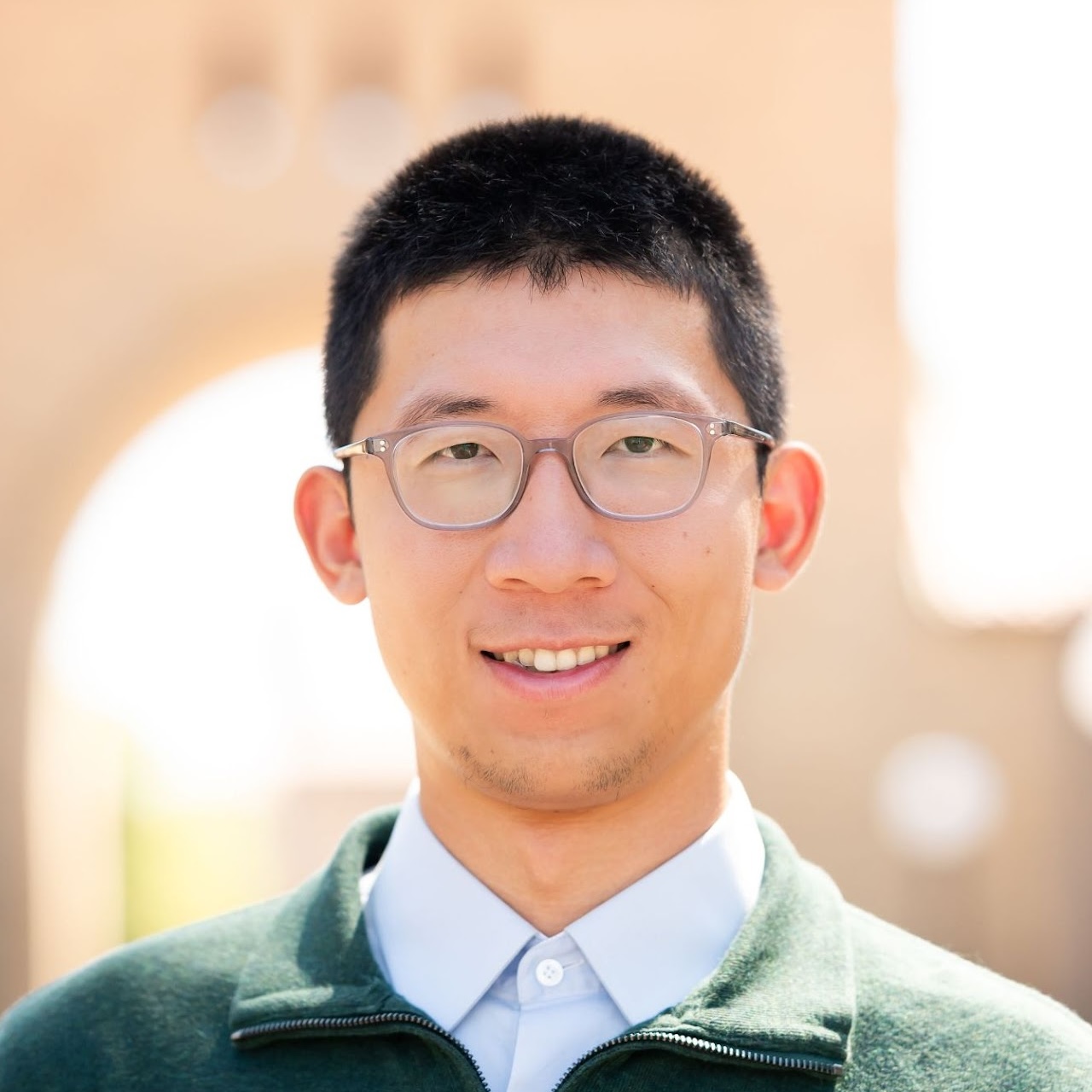
Zhaonan Qu Columbia University
A Distributionally Robust Instrumental Variable Estimation Framework
 
We propose a distributionally robust optimization (DRO) formulation of the classical instrumental variable (IV) regression framework. When the ambiguity set is a Wasserstein ball centered at the empirical distribution of projected variables, the resulting estimator solves a square root version of the standard ridge regularized IV regression. We show that the estimator is consistent whenever the limit of the robustness/regularization parameter is bounded above by an estimable non-zero constant. This novel consistency result differs from existing ones on regularized regression because it allows a non-vanishing regularization parameter. We discuss some interesting features of the problem underlying this phenomenon, namely a projection-based ambiguity set coupled with the unique geometry of the square root ridge regression. We also discuss generalizations to other DRO problems with projection-based ambiguity sets.
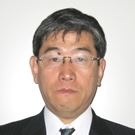
Takashi Tsuchiya GRIPS
Vavasis-Ye algorithm, Information Geometry, and Beyond
 
Vavasis and Ye's layered least squares interior-point algorithm is a polynomial-time interior-point algorithm for LP which runs in \(O(n^{3.5}(\log \bar\chi_A+n))\) iterations to find an exact optimal solution, where \(\bar\chi_A\) is a condition number of \(A\) introduced by Dikin. Since Vavasis and Ye's algorithm is so elegant and fascinating, I studied the algorithm seriously quite some time with my colleagues N.~Megiddo, S.~Mizuno, R.~D.~C.~Monteiro, G.~Lan, S.~Kakihara, A.~Ohara and T.~Kitahara, to make it simple and scaling invariant. In this talk, I reflect this endeavar highlighting an unexpected geometric theorem stemmed from Vavasis and Ye's algorithm bounding total curvature of central trajectories in the framework of information geometry, a discipline of differentional geometry for information science, statistics and machine learning. Recently, a simple scaling invariant variant of Vavaiss and Ye's algorithm was developed by D.~Dadush, Z.~K.~Koh, B.~Natura, N.~Olver and L.~A.~Végh. They accomplished this goal by showing that an approximation of a scaling-invariant two-layer trust-region direction by Monteiro, Tsuchiya and Lan can be computed with enough accuracy in strongly polynomial-time. I also introduce this splendid development with a small side story.
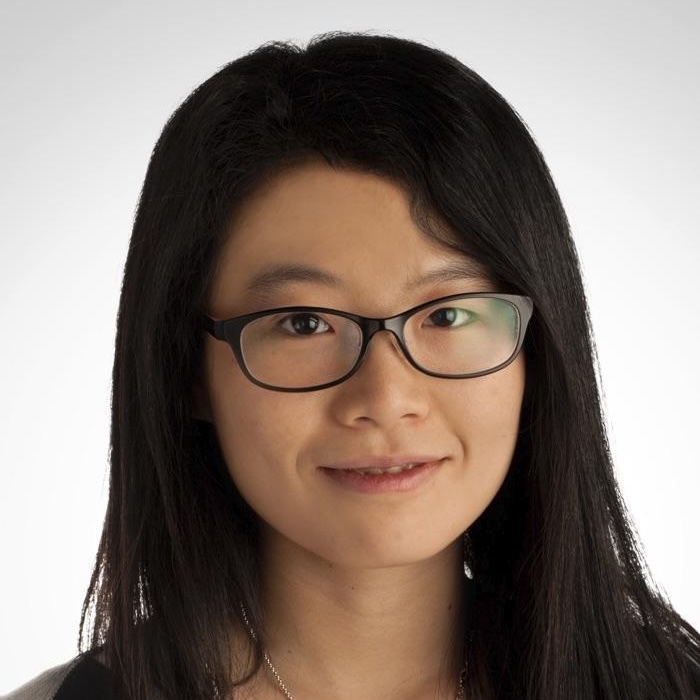
Mengdi Wang Princeton University
Guided Diffusion Models Towards Generative Optimization
 
Diffusion models represent a significant breakthrough in generative AI, operating by progressively transforming random noise distributions into structured outputs, with adaptability for specific tasks through guidance or fine-tuning. In this presentation, we delve into the statistical and optimization aspects of diffusion models and establish their connection to theoretical optimization frameworks. In the first part, we explore how unconditioned diffusion models efficiently capture complex high-dimensional data, particularly when low-dimensional structures are present. We present the first efficient sample complexity bound for diffusion models that depend on the small intrinsic dimension. Moving to the second part, we leverage our understanding of diffusion models to introduce a pioneering optimization method termed "generative optimization." Here, we harness diffusion models as data-driven solution generators to maximize any user-specified reward function. We introduce gradient-based guidance to guide the sampling process of a diffusion model while preserving the learnt low-dim data structure. We show that adapting a pre-trained diffusion model with guidance is essentially equivalent to solving a regularized optimization problem. Further we consider adaptively fine-tuning the score network using new samples together with gradient guidance. This process mimics a first-order optimization iteration, for which we established an O(1/T) converge rate to the global optimum. Moreover, these solutions maintain fidelity to the intrinsic structures within the training data, suggesting a promising avenue for optimization in complex, structured spaces through generative AI.
Group photo of speakers with Yinyu
Coffee available
Session 3 (Chair: Oliver Hinder)
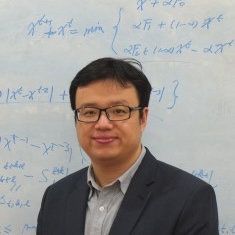
Dongdong Ge Shanghai Jiao Tong University
The Development History of COPT
 
This talk will provide a brief introduction to the development of the COPT mathematical optimization software under the leadership of Professor Yinyu Ye. We will explore its journey from inception to becoming the second best software globally in its field. Additionally, I will share recent advancements in leveraging GPU architectures to accelerate mathematical optimization, a new progress made under his guidance.
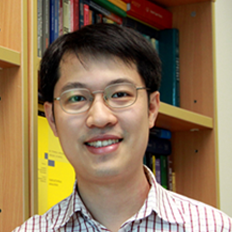
Anthony Man-Cho So Chinese University of Hong Kong
Distance Geometry through the Lens of Optimization
 
A classic problem in distance geometry is that of recovering the positions of a set of objects from an incomplete list of Euclidean distance measurements between pairs of those objects. Although the problem has a simple description, its solution remains challenging and calls for ideas from different branches of mathematics and computer science. In this talk, I share my journey in tackling this problem, which began with my PhD work under Professor Ye's supervision, and discuss how various areas of optimization, including semidefinite optimization, optimization landscape analysis, and manifold optimization, offer powerful tools for understanding both the geometric and algorithmic aspects of the problem.
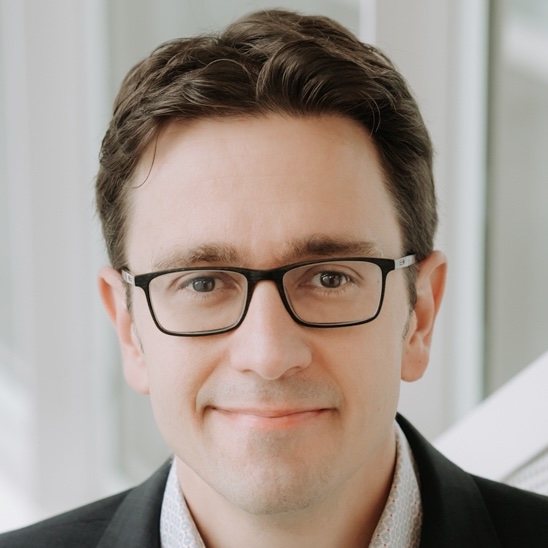
Erick Delage HEC Montreal
Conditional Robust Optimization
 
Conditional Robust Optimization (CRO) is a decision-making framework that blends the flexibility of robust optimization (RO) with the ability to incorporate additional information regarding the structure of uncertainty. This approach solves the RO problem where the uncertainty set structure adapts to account for the most recent information provided by a set of covariates. In this presentation, we will introduce an end-to-end data-driven approach to CRO. We will also show how hypothesis testing can be integrated to the training in order to improve the quality of conditional coverage of the produced uncertainty sets.
Coffee Break
Session 4 (Madeleine Udell)
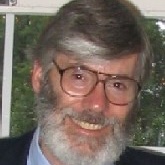
Michael Todd Cornell University
Yinyu Ye's Research on Interior-point Methods
 
We survey Yinyu's early work on potential-reduction and path-following methods and the relationship of these methods to the ellipsoid and simplex methods.
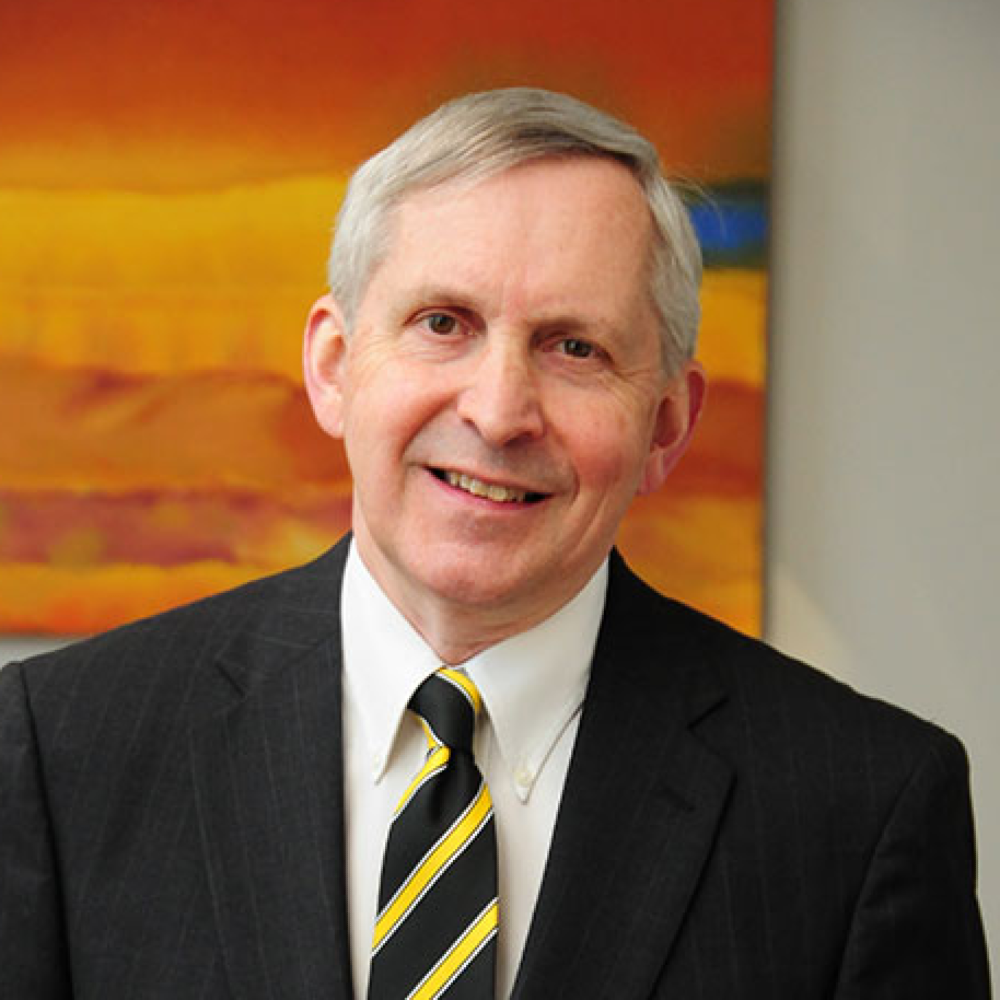
Kurt Anstreicher University of Iowa
More New Results on Quadratic Minimization
 
In their 2003 paper “New results on quadratic minimization”, Ye and Zhang introduced fundamental theory for SDP relaxations applied to extended trust-region subproblems. This paper stimulated a long line of research that continues to this day. In this talk we will describe some positive results that have been obtained as well as open problems that remain.
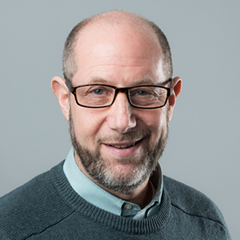
Robert Freund MIT
The Role of Level-Set Geometry on the Performance of PDHG for Conic Linear Optimization
 
In joint work with Zikai Xiong (MIT OR Center), we consider solving huge-scale instances of (convex) conic linear optimization problems, at the scale where matrix-factorization-free methods are attractive or necessary. The restarted primal-dual hybrid gradient method (rPDHG) -- with heuristic enhancements and GPU implementation -- has been very successful in solving huge-scale linear programming (LP) problems. Here we extend the study of rPDHG to encompass Conic Linear Optimization as well. We present a new theoretical analysis of rPDHG for general (convex) conic linear optimization and for LP as a special case thereof. We show a relationship between geometric measures of the primal-dual (sub-)level sets and the convergence rate of rPDHG. We also show how central-path-based linear transformations -- including conic rescaling -- can markedly enhance the convergence rate of rPDHG. Last of all, we present computational results that demonstrate how rescalings can accelerate convergence to high-accuracy solutions, and lead to more efficient methods for huge-scale LP problems in particular. Many of our ideas are aligned with and connected to work by Yinyu Ye, and these connections will be discussed in the talk.
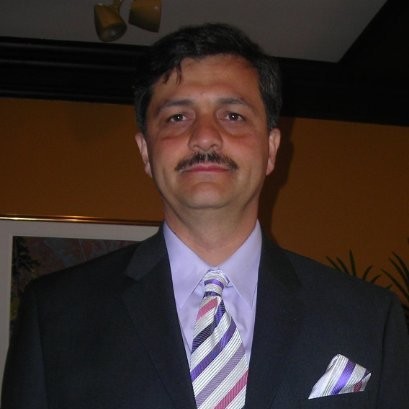
Farid Alizadeh Rutgers University
Using Probabilistic Data in Learning and its Consequences
 
We propose a new approach to machine learning where data, instead of a collection of points in the feature space, are presented in the form of probability distributions over the that space. The motivation for this approach is that observations of data are always inexact, ambiguous, or inherently unsuitable to be presented as an exact point. For instance, for many features, there may be errors in the process of measurement or observation (e.g., someone's weight is recorded as 85kg, but the scale showing this value may be somewhat inaccurate.) Another situation is when the feature being measured is not specific (e.g., the "ethnicity" of a person, which is asked in many employment questionnaires, may be a mixture of values.) In another case, a feature may be a time or spatial series (e.g., a person's blood pressure goes up and down during a day or week.) To better capture these ambiguities, we propose presenting each data line as a (joint) probability distribution over all possible values that an observation could attain. We examine the consequences of such an approach to measuring learning algorithms' performance, likelihood functions, and regression problems. In particular, we show that incorporating the ambiguity in the data through probability distributions results in a natural, "baked-in" regularization in the maximum likelihood regression formula.
Lunch Packard Bytes Cafe or Clark Building
Session 5 (Chair: Zizhuo Wang)
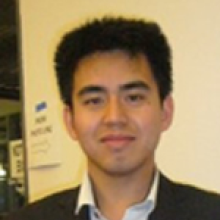
Yichuan Daniel Ding McGill University
Efficiency-Fairness Tradeoff in Service Systems
 
We develop a novel framework that allows us to tight the bound for the price of fairness that was proposed by Bertsimas et al (2011). I will then talk about how efficiency-fairness tradeoffs are made in practical service systems, including the cadaver kidney allocation, affordable housing allocation, emergency department, and an agri-product retailing platform. To make such decisions, the system manager needs to characterize the behaviour of the queueing system, which calls for cutting-edge stochastic modeling and optimization methods.
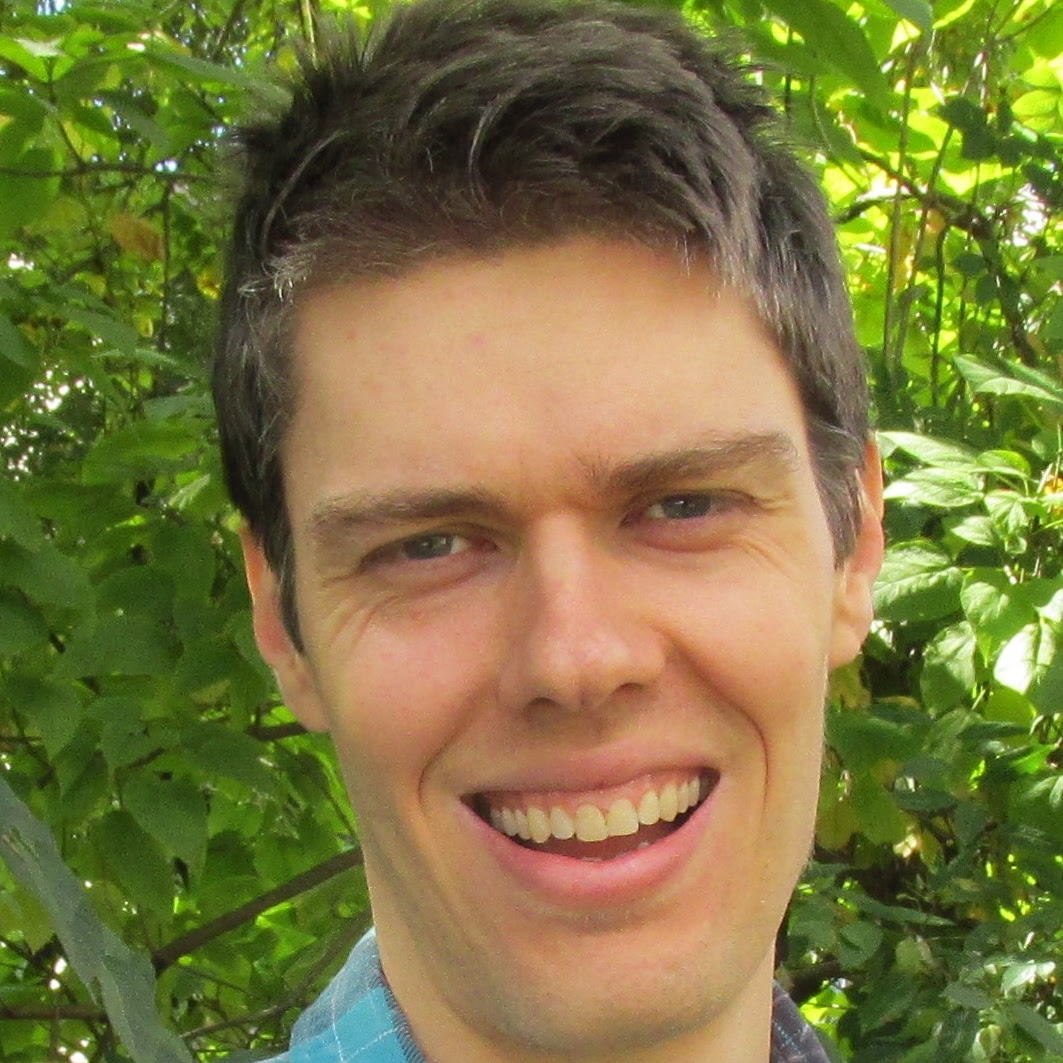
Oliver Hinder University of Pittsburgh
The Price of Adaptivity in Stochastic Convex Optimization
 
We prove impossibility results for adaptivity in non-smooth stochastic convex optimization. Given a set of problem parameters we wish to adapt to, we define a "price of adaptivity" (PoA) that, roughly speaking, measures the multiplicative increase in suboptimality due to uncertainty in these parameters. When the initial distance to the optimum is unknown but a gradient norm bound is known, we show that the PoA is at least logarithmic for expected suboptimality, and double-logarithmic for median suboptimality. When there is uncertainty in both distance and gradient norm, we show that the PoA must be polynomial in the level of uncertainty. Our lower bounds nearly match existing upper bounds, and establish that there is no parameter-free lunch. En route, we also establish tight upper and lower bounds for (known-parameter) high-probability stochastic convex optimization with heavy-tailed and bounded noise, respectively.
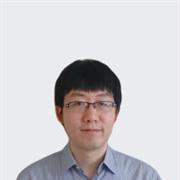
Xiaocheng Li Imperial College London
Watermarking LLMs with Online Linear Programming
 
The watermarking of large language models (LLMs) refers to the procedure of injecting a hidden pattern to the LLM-generated text that is imperceptible to humans but can be algorithmically detected as generated by AI. We consider a natural trade-off of the watermarking procedure between (probability) model distortion and detection ability, and we formulate the watermarking of LLMs as a constrained optimization problem based on the green-red algorithm of Kirchenbauer et al. (2023). We show that the optimal solution to the optimization problem enjoys a nice analytical property. This analytical structure renders the formulation fall under the framework of online optimization with constraints where the existing online linear programming algorithms in OR/MS literature can be naturally applied. These developments also shed light on the properness of model distortion metrics and establish an interesting connection between the repetition phenomenon for the LLM-generated texts and the dual variable of the online optimization problem.
Coffee Break
Session 6 (Chair: Mike Todd)
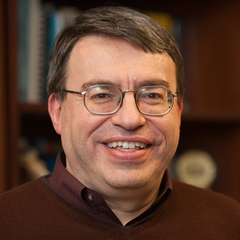
Dimitris Bertsimas MIT
Global Optimization for Classes of Non-convex Problems
 
Yinyu made substantial contributions in approximating non-convex problems. In this work, we propose a new global optimization approach for solving non-convex optimization problems in which the non-convex components are sums of products of convex functions. A broad class of non-convex problems can be written in this way, such as concave minimization problems, difference of convex problems, and fractional optimization problems. Our approach exploits two techniques: first, we introduce a new technique, called the Reformulation-Perspectification Technique (RPT), to obtain a convex approximation of the considered non-convex continuous optimization problem. Next, we develop a spatial Branch and Bound scheme, leveraging RPT, to obtain a global optimal solution. Numerical experiments on several different convex maximization problems, a quadratic constrained quadratic optimization problem, and a dike height optimization problem demonstrate the effectiveness of the proposed approach. In particular, our approach solves more instances to global optimality for the considered problems than BARON and SCIP. We further discuss extensions to robust and adaptive optimization problems. (Joint work Danique de Moor, Dick den Hertog, Thodoris Koukouvinos, Jianzhe Zhen).
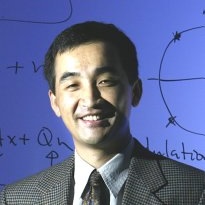
Tom Luo CUHK, Shenzhen
Proximal ADMM for Non-convex Problems
 
We propose a proximal alternating direction method of multipliers to solve a linearly constrained nonconvex minimization problem. At each iteration, the algorithm first inexactly minimizes a proximal augmented Lagrangian function and then updates the dual multiplier vector using the residual of the linear constraints. When the primal and dual stepsizes are chosen sufficiently small, we show the iterates of the resulting proximal ADMM algorithm converges to a stationary point of the nonconvex problem, provided some mild regularity conditions are satisfied. When the objective function is quadratic, we further establish the linear convergence of the algorithm.
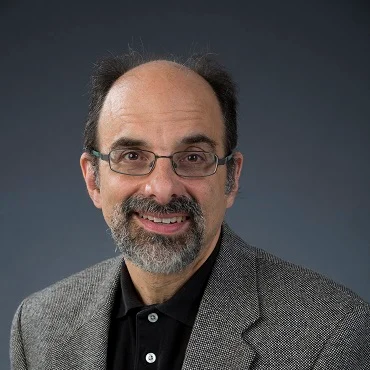
Stephen Vavasis University of Waterloo
First-order Methods for Linear Programming
 
Linear programming has been conventionally solved with either the Simplex method or interior-point methods. In the past 10 years, first-order methods, which use only matrix-vector multiplication, have emerged as a competitor to the conventional methods for very large problems. In this talk, I will survey some of these methods including ADMM and PDHG, in which Yinyu Ye has made important advances.
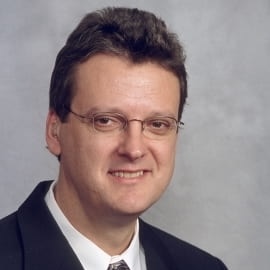
Renato Monteiro Georgia Institute of Technology
A Low-Rank Augmented Lagrangian Method for Large-Scale Semidefinite Programming Based on a Hybrid Convex-Nonconvex Approach
 
This talk discusses the theory of a new first-order method for solving large-scale semidefinite programs (SDPs) with bounded domain. It is an inexact augmented Lagrangian (AL) method where the AL subproblems are solved by a novel hybrid low-rank method. In contrast to the classical low-rank method, the new method finds a near-optimal solution (with provable complexity bounds) of SDP instances satisfying strong duality. Computational results (to be presented in the next talk) comparing the new method to state-of-the-art solvers on several large SDP instances show that the former finds higher accurate solutions in substantially less CPU time than the latter ones. For example, it can solve in less than 30 minutes, and \(10^{-5}\) relative error condition, a maximum stable set SDP with a \(10^6\times10^7\) matrix variable. Co-authors: Arnesh Sujanani and Diego Cifuentes
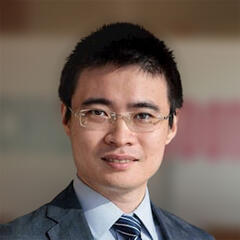
Haihao Lu MIT
GPU-Accelerated Linear Programming
 
Linear Programming is a fundamental tool in operations research and computational science that has been widely used in practice. This is a field that Yinyu has made propounding contributions, particular the theory and computation of interior-point method. In this talk, we will discuss a new class of algorithms for linear programming, and how GPU architectures can accelerate the convergence performance. This line of research includes recent collaboration with Yinyu.